Gavin Schmidt asks at RealClimate: “How should one make graphics that appropriately compare models and observations?” and goes on to reconstruct John Christy’s updated comparison between climate models and satellite temperature measurements. The reconstruction was cited here by Simon in response to Gary Kerkin’s reference to Christy’s graph ( – h/t Richard Cumming, Gary Kerkin and Simon for references).
There’s been a lot of blather about Christy’s telling graph and heavy criticism here from Schmidt — but the graph survives. In taking all the trouble to point out where Christy is wrong, even going so far as to provide an alternative graph, Schmidt amazingly fails to alter the impression gained from looking at it. Even in his reconstruction the model forecasts still soar way above the observations.
So Christy’s graph is true. What else does it need to say to sentence the models to fatal error and irrelevance? Are our policy makers listening?
Though he alters the baseline and other things, the graphic clearly reveals that since about 1998 most climate models have continued an excursion far above the actual surface temperatures. This failure for nearly 20 years to track actual temperatures reveals serious faults with the models and strongly suggests the greenhouse hypothesis itself is in deep trouble.
The continued refusal of those in charge of the models to announce that there’s a problem, to talk about it or explain what they’re doing to fix it, illustrates the steadfastly illusory nature of the alarm the models — and only the models — underpin. When the models are proven wrong like this yet tales of alarm don’t stop, we can be sure that the alarm is not rooted in reality.
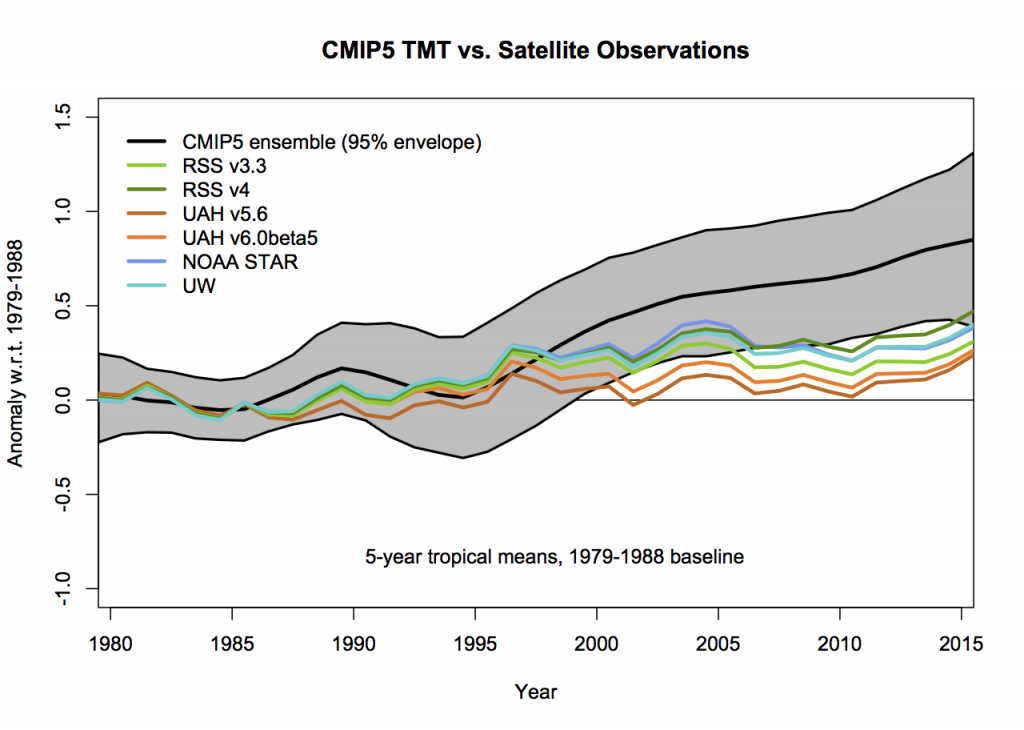
Gavin Schmidt’s ‘improved’ version of John Christy’s iconic graph comparing climate model output with real-world temperatures
Here’s Christy’s updated graph, as he presented it to the U.S. House Committee on Science, Space & Technology on 2 Feb 2016.
Gavin Schmidt’s confirmation of the essence of Christy’s criticism of the models signals deep problems with Schmidt’s continued (stubborn?) reliance on the models. Christy’s presentation goes on:
The information in this figure provides clear evidence that the models have a strong tendency to over-warm the atmosphere relative to actual observations. On average the models warm the global atmosphere at a rate 2.5 times that of the real world. This is not a short-term, specially-selected episode, but represents the past 37 years, over a third of a century. This is also the period with the highest concentration of greenhouse gases and thus the period in which the response should be of largest magnitude.
Richard Cumming has several times recently said (on different grounds) that “we are witnessing the abject failure of the anthropogenic global warming theory.” I can only agree with him.
Views: 619
The El Nino spike at the beginning of 2016 was as good as it gets for Schmidt, now he’s looking at his own GISTEMP cooling rapidly. That was the El Nino Schmidt claimed for man-made climate change leaving the El Nino contribution only 0.07 C:
Egg on face now.
>”Christy comments that “the Russian model (INM-CM4) was the only model close to the observations.”
I inquired from John what that model was when he first started compiling these graphs. He didn’t know why INM-CM4 was different. Others have looked into it though:
INMCM4 (Russian Academy of Sciences) in Judith Curry’s post: ‘Climate sensitivity: lopping off the fat tail’
There is one climate model that falls within the range of the observational estimates: INMCM4 (Russian). I have not looked at this model, but on a previous thread RonC makes the following comments.
“On a previous thread, I showed how one CMIP5 model produced historical temperature trends closely comparable to HADCRUT4. That same model, INMCM4, was also closest to Berkeley Earth and RSS series.
Curious about what makes this model different from the others, I consulted several comparative surveys of CMIP5 models. There appear to be 3 features of INMCM4 that differentiate it from the others.”
1.INMCM4 has the lowest CO2 forcing response at 4.1K for 4XCO2. That is 37% lower than multi-model mean
2.INMCM4 has by far the highest climate system inertia: Deep ocean heat capacity in INMCM4 is 317 W yr m22 K-1, 200% of the mean (which excluded INMCM4 because it was such an outlier)
3.INMCM4 exactly matches observed atmospheric H2O content in lower troposphere (215 hPa), and is biased low above that. Most others are biased high.
So the model that most closely reproduces the temperature history has high inertia from ocean heat capacities, low forcing from CO2 and less water for feedback.
Definitely worth taking a closer look at this model, it seems genuinely different from the others.
http://judithcurry.com/2015/03/23/climate-sensitivity-lopping-off-the-fat-tail/
Also, from Stephens et al (2012) :
Figure 2a The change in energy fluxes expressed as flux sensitivities (Wm−2 K−1) due to a warming climate.
(top graph – TOA)
http://www.nature.com/ngeo/journal/v5/n10/fig_tab/ngeo1580_F2.html
For all-sky OLR, INM-CM4 is the only model with a positive sensitivity (system heat gain). It also has the highest positive clear sky OLC-C sensitivity (heat gain).
Basically, INM-CN4 is the only model exhibiting system heat gain from an outgoing longwave radiation forcing.
‘Climate Models are NOT Simulating Earth’s Climate – Part 4’
Posted on March 1, 2016 by Bob Tisdale
Alternate Title: Climate Models Undermine the Hypothesis of Human-Induced Global Warming
[…]
More>>>>>
https://bobtisdale.wordpress.com/2016/03/01/climate-models-are-not-simulating-earths-climate-part-4/
# # #
The earth’s energy balance is the IPCC’s primary criteria for climate change whether natural cause or anthropogenic theory. A positive imbalance is a system heat gain, a negative imbalance is a system heat loss. But contrary to IPCC’s climate change criteria, even the climate models exhibiting a negative imbalance also exhibit a system heat gain.
The IPCC omitted to mention this in AR5 Chapter 9 Evaluating Climate Models i.e. it is not necessarily what the IPCC says that matters, the biggest issues lie in what they don’t say (is that incompetent negligence or willful negligence?).
It was left to Bob Tisdale to bring the issue to light. Thanks Bob.
!
Wow. Thanks for highlighting this. We also have it on the very good authority of M. Mann that the models don’t replicate earth’s climate. Multiple streams of evidence now invalidate the models.
>”The continued refusal of those in charge of the models to announce that there’s a problem,……”
To be fair, the IPCC announced the problem in Chapter 9 Box 9.2. They even offer 3 or 4 reasons why the models are wrong. Main ones are that model sensitivity to CO2 is too high (CO2 forcing is excessive) and neglect of natural variation. The latter what sceptcs had been saying for years previous to AR5 and the IPCC finally had to concede.
We didn’t read the news headlines about that though.
Thing is, if the smoothed observation data does not rise above flat over the next 3, 4, 5, 6, 7 years (give them 5 yrs), CO2 forcing is not just excessive, it is superfluous.
This is the acid test for the GCMs. The AGW theory having already failed its acid test by the IPCC’s own primary climate change criteria (earth’s energy balance measured at TOA), see this article for that:
IPCC Ignores IPCC Climate Change Criteria – Incompetence or Coverup?
https://dl.dropboxusercontent.com/u/52688456/IPCCIgnoresIPCCClimateChangeCriteria.pdf
Yes, good points. Of course I’d seen the AR5 comments but the penny didn’t drop when I was writing this — though there’s still been no rush to a press conference to announce an investigation. I’m still coming to grips with that “IPCC ignores” article.
>”…..there’s still been no rush to a press conference to announce an investigation”
No investigation but at least someone is trying to take natural multi-decadal variation (MDV) out of the models -obs comparison. I’ve intoned on this here at CCG at great length so not much here. Basically, models don’t model ENSO or MDV so both must be removed from observations before comparing to model runs.
Kosaka and Xie are back on this after their first attempt which I thought was reasonable (I think I recall), I would point out that there is already a body of signal analysis literature that extracts the MDV signal from GMST – it’s not that difficult. What happens then though is that there’s a miss-attribution of the residual secular trend (ST, a curve) to the “anthropogenic global warming signal”. It becomes obvious that the ST is not CO2-forced when the ST is compared to the CO2-forced model mean. The model mean is much warmer than the ST in GMST.
Problem now is: Kosaka and Xie’s latest effort below is nut-case bizarre:
‘Claim: Researchers create means (a model) to monitor anthropogenic global warming in real time’
https://wattsupwiththat.com/2016/07/18/claim-researchers-create-means-a-model-to-monitor-anthropogenic-global-warming-in-real-time/
This is their graph:
[Please excuse my shouting here]
THEY END UP WITH MORE agw WARMING THAN THE ACTUAL OBSERVATIONS FROM 1995 ONWARDS
Shang-Ping Xie says this:
No Shang-Ping, the greenhouse gas warming is grossly over estimated, including in your new method.
Kosaka and Xie are either astoundingly stupid themselves, or they think everyone else is.
From 1980 on, Kosaka and Xie’s graph is no different to Schmidt’s or Christy’s.
–
Confirmation bias makes them forget the supremacy of data over hypothesis.
You should probably explain to casual readers what TMT actually is, as it is not obvious.
Temperature measurements in the mid-troposphere are considerably less understood than the surface.
Note that the CMIP5 runs are projections not predictions. The comparison would have been much fairer if the models were retrospectively re-run with known solar + volcanic forcings and ENSO outcomes.
There is as yet insufficient evidence to say that the models are biased and there may yet be issues with satellite observation degradation.
Simon,
Well, you’ve just done so; thanks. Whether you call them predictions or projections doesn’t matter—they were wrong. Volcanic forcings I agree with. But the fact that models cannot predict solar variation or ENSO influences is very significant, for without those, you’ll never have an accurate picture of our temperatures, wouldn’t you say? You can scarcely say “we don’t do ENSO” and still expect us to uproot all the energy sources we rely on for our prosperity.
Insufficient evidence to show model bias? That’s not the point; there’s plenty of evidence that they’re wrong. I’m not too worried about satellite degradation; if they prove unreliable, the balloon observations will do.
I’m still interested in your response to my questions about the papers cited by Renwick and Naish in the slide featured in the previous post:
There is a big difference between a projection and a prediction. We can predict the weather a few days out, but a projection of what a company or technology might look like in 10 years time is largely speculation and guesswork.
Simon
>”The comparison would have been much fairer if the models were retrospectively re-run with known solar + volcanic forcings and ENSO outcomes.”
Heh. Except for ENSO (wrong there – see below) you’re just repeating what the IPCC conceded in AR5 Chapter 9 Box 9.2. But they add that CO2 sensitivity is too high i.e. CO2 forcing is excessive, and that “natural variation” (not ENSO) has been neglected. In effect, you are tacitly admitting the models are wrong Simon. You’re not alone, the IPCC do too.
Volcanics are transitory – see below.
ENSO is irrelevant and NOT the “natural variation” the IPCC are referring too. Models don’t do ENSO. Therefore a direct models-obs comparison is with ENSO noise smoothed out. When Schmidt does that in his graph (as Christy did) he’ll be 100% certain that 95% of the models are junk.
“Natural variation” is predominantly due to oceanic oscillations e.g. AMO, PDO/IPO etc, otherwise known as multi-decadal variation (MDV), which distort the Northern Hemisphere temperature profile which then overwhelms GMST. The oscillatory MDV signal is close to a 60 yr trendless sine wave which can be subtracted from GMST so that the residual secular trend (ST) can be compared to to the model mean in Schmidt’s and Christy’s graphs.
GMST – MDV = ST
Problem is: the model mean will never conform to the GMST secular trend (ST) as long as the models are CO2 forced. The GMST ST passes through ENSO-neutral data at 2015 from BELOW. 2015 is a crossover date when MDV goes from positive to negative i.e. it is on the MDV-neutral “spline”.
MDV-neutral “spline” in GMST:
2045 neutral
2030 < MDV max negative
2015 neutral………………………(CO2 RF 1.9 W.m-2 @ 400ppm, ERF 2.33+ W.m-2)
2000 < MDV max positive
1985 neutral
1970 < MDV max negative
1955 neutral………………………(CO2 forcing uptick begins)
1940 < MDV max positive
1925 neutral
1910 < MDV max negative
1895 neutral
Schmidt and Christy's graphs are normalized at the beginning of the satellite era so cannot be used in this exercise. The IPCC shows models vs HadCRUT4 and it is perfectly clear that the models CONFOM do the MDV-neutral spline up until the CO2 forcing uptick kicks in at MDV-neutral 1955.
From IPCC AR5: CMIP3/5 models vs HadCRUT4 1860 – 2010
https://tallbloke.files.wordpress.com/2013/02/image1.png
After 1955, the model mean DOES NOT CONFORM to the MDV neutral spline. Volcanics distort the picture 1955 – 2000 but easy to see that at 2000 when MDV is max positive, the model mean corresponds to observations – it SHOULD NOT. The model mean should be BELOW observations at 2000. Remember that the MDV signal must be subtracted from observations (GMST) in order to compare directly to models.
Volcanics are transitory so can be ignored in this exercise i.e. just connect the model mean at the end of volcanic distortion (2000) to the mean before the volcanics took effect (1955). Similarly observations are distorted by volcanics but only temporarily.
So now we can correct your statement Simon:
Correct now.
I absolutely support the general conclusions of these studies. The proportion of climate scientists who support the AGW hypothesis is greater than 95%. Do you concur?
I am most curious how an non-specialist such as yourself can somehow ‘know the truth’ whereas the scientific consensus has ‘got it wrong’. You might also like to comment how you managed to avoid the Dunning-Kruger effect.
Note also that ENSO, like weather, is chaotic. We can predict the probability of occurrence, but not when they will occur beyond a limited time horizon. What is important is the trend. An expert would know that.
Nobody apart from yourself is talking about ‘uprooting energy sources’. I would suggest that it is this concern that is causing your cognitive bias.
‘A TSI-Driven (solar) Climate Model’
February 8, 2016 by Jeff Patterson
“The fidelity with which this model replicates the observed atmospheric CO2 concentration has significant implications for attributing the source of the rise in CO2 (and by inference the rise in global temperature) observed since 1880. There is no statistically significant signal of an anthropogenic contribution to the residual plotted Figure 3c. Thus the entirety of the observed post-industrial rise in atmospheric CO2 concentration can be directly attributed to the variation in TSI, the only forcing applied to the system whose output accounts for 99.5% ( r2=.995) of the observational record.
How then, does this naturally occurring CO2 impact global temperature? To explore this we will develop a system model which when combined with the CO2 generating system of Figure 4 can replicate the decadal scale global temperature record with impressive accuracy.
Researchers have long noted the relationship between TSI and global mean temperature.[5] We hypothesize that this too is due to the lagged accumulation of oceanic heat content, the delay being perhaps the transit time of the thermohaline circulation. A system model that implements this hypothesis is shown in Figure 5.”
“The results (figure 10) correlate well with observational time series (r = .984).”
http://wattsupwiththat.com/2016/02/08/a-tsi-driven-solar-climate-model/comment-page-1/
# # #
Goes a long way towards modeling Multi-Decadal Variation/Oscillation (MDV/MDO).
Long system lag (“oceanic delay”), well in excess of 70 years depending on TSI input series.
Cannot be accused of “curve fitting” (but was in comments even so).
Models CO2 as an OUTPUT. The IPCC’s climate models parameterize CO2 as an INPUT.
The proportion of climate scientists who support the AGW hypothesis is greater than 95%.
What is the AGW hypothesis?
What hypothesis, or lack thereof, do the other 3-5% subscribe to, to explain whatever phenomenon they assert needs explaining?
Simon
>”I absolutely support the general conclusions of these studies. The proportion of climate scientists who support the AGW hypothesis is greater than 95%. Do you concur?”
Climate scientists? I don’t think so.
From Verheggen (2014):
An “eco-Marxist analysis” is a scientific analysis of global warming by climate scientists?
Get real Simon.
Similarly for Cook et al. Psychologist José Duarte writes:
“Psychology studies, marketing papers, and surveys of the general public” are scientific analyses of global warming by climate scientists?
Get real Simon.
Andy
>”What is the AGW hypothesis?”
Has never been formally drafted. But we can infer one from the IPCC’s primary climate change criteria:
Falsified by the IPCC’s cited observations in AR5 Chapter 2.
Simon,
You err in supposing I “know the truth”. I simply point out errors in some of these studies, whether those errors have been discovered by others or myself. It’s obvious you have no interest in facing those errors, as you offer no comment about them, and that’s curious. Aren’t you keen to know the truth?
What consensus? There’s no evidence, given the grievous errors in some of these studies, of a substantial consensus. You’re the one with a cognitive bias, Simon.
How do you know that I did? But that’s for others to comment on— how could I know?
Sorry, Simon, this was roughly expressed. I meant to say replacing traditional, reliable energy sources such as coal, oil and gas responsible for our prosperity with modern wind turbines, solar panels, thermal solar plants, tidal and wave power generation and burning North American wood chips in UK generating stations, while steadfastly avoiding the two leading contenders for emissions-free electricity: hydro and nuclear. Not to mention solving the horrendous scheduling problems of generating with fickle breeze-blown windmills and solar panels that go down every night.
In brief: uprooting traditional energy sources.
I meant to say that others certainly are advocating ripping out traditional energy sources. You could start with the Coal Action Network with their slogan “keep the coal in the hole” and a campaign for Fonterra to quit coal and move on to examine the WWF, Greenpeace and the UN. All of those want to end our industrial practices for the sake of the planet.
Simon
>”What is important is the trend.”
No Simon, the (temperature) trend is not important. It is not even critical. What is critical is the IPCC’s primary climate change criteria:
Crud. Missed a tag after “The energy balance of the Earth-atmosphere system…..measured at the top of the atmosphere”
I Was using my “slim”, but fast, browser version which doesn’t give me the Edit facility.
>”The oscillatory MDV signal is close to a 60 yr trendless sine wave which can be subtracted from GMST so that the residual secular trend (ST) can be compared to to the model mean…..”
Bob Tisdale doesn’t grasp this. See his latest:
‘June 2016 Global Surface (Land+Ocean) and Lower Troposphere Temperature Anomaly Update’
Bob Tisdale / July 19, 2016
https://wattsupwiththat.com/2016/07/19/june-2016-global-surface-landocean-and-lower-troposphere-temperature-anomaly-update/
Scroll down to MODEL-DATA COMPARISON & DIFFERENCE
Tisdale doesn’t grasp that 1910 was max negative MDV (see GMST “spline” from upthread reproduced below). Of course the models will be at +0.2 above the observations at 1910. The MDV signal must be subtracted from GMST in order to compare to the model mean. Once that’s done the model mean conforms to the MDV-neutral GMST “spline” at 1910.
MDV-neutral “spline” in GMST:
2045 neutral
2030 < MDV max negative
2015 neutral………………………(CO2 RF 1.9 W.m-2 @ 400ppm, ERF 2.33+ W.m-2)
2000 < MDV max positive
1985 neutral
1970 < MDV max negative
1955 neutral………………………(CO2 forcing uptick begins)
1940 < MDV max positive
1925 neutral
1910 < MDV max negative
1895 neutral
At MDV-neutral 1925 no subtraction of the MDV signal is needed from GMST. At that date the model mean and observations correspond exactly.
Similar to 1910 but opposite is 1940 which was max positive MDV. Of couse the models will be at -0.1 below the observations. The MDV signal must be subtracted from GMST in order to compare to the model mean. Once that's done the model mean conforms to the MDV-neutral GMST "spline" at 1940.
At MDV-neutral 1955 no subtraction of the MDV signal is needed from GMST. At that date the model mean and observations correspond exactly.
After 1955 the picture is distorted by transitory volcanics which Tisdale has "minimised" by 61 month smoothing but there is still much distortion. The cycle prior to 1955 is broken up.
At MDV-neutral 1985 the models and observations correspond but that is illusory given the volcanic distortion. Still, it is indicative.
At max MDV positive in 2000, The models SHOULD be BELOW observations BUT THEY ARE NOT. They are +0.05 above observations.
At MDV-neutral 2015 no subtraction of the MDV signal is needed from GMST. But at that date instead of the model mean and observations corresponding exactly as back in 1925 1955 and 1985 (sort of), the models are +0.17 above observations.
When data crunchers like Bob Tisdale can't grasp the models-obs apples-to-apples necessity of subtracting the MDV signal from GMST, it is going to take a long long time before the real models-obs discrepancy is realized.
That discrepancy only begins 1955 (1985 notwithstanding) and is due to CO2 forcing from 1955 onwards.
Michael Mann:
Apparently Michael Mann “supports the AGW hypothesis” (as per Simon), but he’s having a great deal of difficulty actually applying it to the real world.
That’s if his use of the phrase “trying to tease out” is anything to go by.
I am not a scientist of any kind, but learned many years ago that data gained from careful and thorough observation trumps models every time.
While I have earned a living doing many things at various times, I have found that engineers work from a philosophy that I can agree with. Scientists not so much, particularly ‘Climate Scientists’.
Schmidt’s latest:
No mention of what the “other factors” are. Note the plural.
And no mention that the temperature spike peaking in Feb 2016 was only in the Northern Hemisphere.
Richard C. “When it comes to what caused the record highs of 2016, “we get about 40 per cent of the record above 2015 is due to El Nino, and 60 per cent is due to other factors”, Schmidt said.”
When I eyeball the graphs presented by Christy and Spencer it looks to me as though the fractions are the other way round i.e. 40% is due to other factors and 60% is due to El Niño. Seems to be equally true of both 1998 and 2015.
“Other factors” means, I presume, those factors which have caused the rise in temperature since the Little Ice Age.
I have been watching this thread throughout the day but because I’ve been trotting backwards and forwards from Hutt Hospital experiencing the modern technological miracle of a camera capsule which has been peering at the inside of my gut, it is only now that I’ve had a chance to comment.
A couple of points seem very clear to me. One is that associated with trying to compare reality with simulations and, in particular the Schmidt criticisms of the comparisons of Christy and Spencer. I commented on this in the previous post, highlighting the carping nature of the criticisms. These have been reinforced by the comments of both Richards today. As has been pointed out, even if the parameters are manipulated as described by Schmidt, we are still left with the indisputable conclusion that the output of the models do not match reality. This has also been commented on by Tisdale, as pointed out by Richard C. Despite what RC sees as deficiencies in Tisdale’s number crunching, the difference is clear.
The other point relates to semantics. Andy comments that there is a difference between “projection” and “prediction”. If you Google the terms together you’ll get 26,000,000+ results. A cursory look at some that try to draw a distinction yields little useful information. As far as I can see the terms are used very loosely and I think that is really because they mean much the same thing. For example a dictionary definition of “projection” is “an estimate or forecast of a future situation based on a study of present trends”, and a dictionary definition of “prediction” is “a thing predicted; a forecast”. Can you really identify a difference between the two definitions? I can’t.
The IPCC chooses to draw the following distinction (http://www.ipcc-data.org/guidelines/pages/definitions.html):
“Projection”
“The term ‘projection” is used in two senses in the climate change literature. In general usage, a projection can be regarded as any description of the future and the pathway leading to it. However, a more specific interpretation has been attached to the term ‘climate projection” by the IPCC when referring to model-derived estimates of future climate.”
“Forecast/Prediction”
“When a projection is branded ‘most likely’ it becomes a forecast or prediction. A forecast is often obtained using deterministic models, possibly a set of these, outputs of which can enable some level of confidence to be attached to projections.”
So the IPCC has tried to change the definition of “projection” so that it is tied to the models and it has said it will become a “prediction” when the “projection” is the most likely outcome.
There seems to me to be something slightly oxymoronic in this and I really have to wonder if it isn’t an attempt to obfuscate what they are portraying. Is there anyone who doesn’t believe that weather forecasts (read “predictions”) are not now made without the assistance of computer models? In what way, then, do they differ in nature from predictions made from climate models? Other than the latter are based on an hypothesis of AGW and with assumptions which, from the comparisons discussed here, are not exactly up to snuff, while the former are presumably based on atmospheric physics and, probably, linear or non-linear modelling of the parameters associated with the atmospheric physics.
Can we now claim the new oxymoron “climate model”?
Gary >“Other factors” means, I presume, those factors which have caused the rise in temperature since the Little Ice Age.
Yes those, of which Schmidt is wedded to AGW, but I think he’s alluding to other phenomena too which are the greater influence in this case I think and Schmidt seems to think too.
When Gareth Renowden posted his February 2016 “wakeup call” post, Andy S and I both looked at the latitudinal breakdown in the Northern Hemisphere and said there’s some research needed to be done because the spike was minimal to non-existent around the NH tropics but from the NH extratropics to the NH polar latitudes the spike just got greater and greater. That’s this graph:
GISTEMP LOTI February 2016 mean anomaly by latitude
http://l2.yimg.com/bt/api/res/1.2/ABpZjd4AnHFp.4g2goHRfg–/YXBwaWQ9eW5ld3NfbGVnbztxPTg1/http://media.zenfs.com/en-US/homerun/mashable_science_572/bb88a1a459ed8b467290bac3540e39dd
Sometimes that URL gives a browser error but I’ve just checked and got the graph up so shouldn’t be a problem (try ‘Open in another tab’).
I’m sure you will agree that the graph is extraordinary. There must be much more than just an El Nino effect. I’m guessing something like AMO or Arctic Oscillation (AO a.k.a. Northern Hemisphere annular mode). But I really don’t know what the driver of that spike is except that I think the El Nino effect was the smaller factor. I’m actually inclined to agree with Gavin Schmidt’s assessment (as far as I’ve quoted him above anyway) in respect to something like 40% El Nino and 60% “other factors” e.g. NH annular mode.
Obviously though, AGW was not a factor (see graph from 55 South to 90 South – no AGW there). Hopefully some climate scientists will present something on it eventually.
Richard C, yes it does look quite extraordinary. I assume there is a strong seasonal component, i.e. the same plot for, say, July, would be something like a mirror image? Did you and Andy look at all months, or just February?
My comment about relative attribution was based merely on eyeballing the temperature graph and looking at the size of the spike compared to where some sort of average would pass beneath it. I have to say that I try to keep it very simple. So much of the information is buried in what I can only refer to as noise that I find it hard to believe than anything useful can be extracted from the data. Even using the most sensitive type of filtering (Kalman, say) I would have difficulty in believing anything that came out of it. GIGO?
[IPCC] >“When a projection is branded ‘most likely’ it becomes a forecast or prediction”
“Branded”?
This is the ultimate in confirmation bias by whoever does the branding. If the forecast scenario is a “description of the future and the pathway leading to it” then it is merely speculation whatever “branding” is assigned to it.
The forecast only becomes ‘most likely’ when it tracks reality i.e. regular checks like those of Christy and Schmidt are needed to see how the forecast is tracking. When a forecast doesn’t track reality it is useless, not fit for purpose, a reject in quality control terms. This is the scientific equivalent of industrial/commercial key performance indicators (KPIs) or budget variances.
The only purpose 95% of the models at mid troposphere (97% at surface) have now is to demonstrate that the assumptions they are based on are false.
We should challenge the MfE, NIWA, and Minister for Climate Change Paula Bennett to post the IPCC’s forecasts graphed against real world atmospheric temperature (also sea levels) and updated as new data comes in. All MfE and NIWA do is state forecasts, there is never any governmental check of those forecasts against reality.
Richard C >”We should challenge the MfE, NIWA, and Minister for Climate Change Paula Bennett to post the IPCC’s forecasts graphed against real world atmospheric temperature (also sea levels) and updated as new data comes in”
They might do it, but I doubt it. I rather feel they would want to restrict themselves to NZ only at best. At worst they would just ignore it. The PCE did but only succeeded in opening herself up to the criticism that the IPCC prognostications on sea level most certainly do not apply to NZ.
The government can quite rightly claim that it is not within its purview to “check” the correctness or otherwise of agency forecasts. The forecasts are published regularly and anyone with the desire to find out for themselves can compare reality with forecast. I suppose a comparison of forecast with reality could be included in the kpi’s of an agency, the outcome of which could influence future funding. But that is another can of worms!
Gary >”Did you and Andy look at all months, or just February?”
No unfortunately. I picked up that graph from a comment thread somewhere. I don’t where to access the original graphs. I know there are similar graphs of each month because I saw another one recently of May I think it was but I didn’t save the link. I wish I knew who produces them. It’s not GISS I don’t think. I suspect it’s someone at Columbia University because the GISTEMP Graphs page links to Columbia:
Columbia: Global Temperature — More Figures
http://www.columbia.edu/~mhs119/Temperature/T_moreFigs/
About half way down there’s this latitudinal breakdown:
Regional Changes – Zonal Means
Zonal mean, (a) 60-month and (b-d) 12-month running mean temperature changes in five zones: Arctic (90.0 – 64.2°N), N. Mid-Latitudes (64.2 – 23.6°N), Tropical (23.6°S), S. Mid-Latitudes (23.6 – 64.2°S), and Antarctic (64.2 – 90.0°S). (Data through June 2016 used. Updated on 2016/07/19, now with GHCN version 3.3.0 & ERSST v4)
http://www.columbia.edu/~mhs119/Temperature/T_moreFigs/ZonalT.gif
Perfectly clear in (a) that the Arctic skews the entire global mean.
Perfectly clear in (b) that the Antarctic is going in the opposite direction to the Arctic.
Perfectly clear in (c) that the Northern Hemisphere is the overwhelming influence in the global mean.
In other words, the global mean is meaningless.
Richard C>”In other words, the global mean is meaningless.”
Yes and/or no, Richard.
My guess is that the skew on those graphs is, as I implied earlier, a seasonal factor and, as I stated, July should almost be a mirror image of February. That implies that that seasonality should be removed and the simplest way of doing that is to average over a year. The shape of the graph if all values were averaged over 12 months would show the underlying skewness applying to either pole.
There are some (Vincent Gray, for example) that argue that a global average is meaningless. My view is that a number of some sort has to be generated if any sort of comparison is to be made. But (and it is a big “but”) having generated an average which contains both geographic and time components, we need to be extremely circumspect about how we use it and what conclusions we may draw from it. Especially if variations in time sit in what is obviously noise!
“The global mean is meaningless.” What do you mean? It is a useful metric.
The important thing is that reality is panning out just as the models predicted. The Arctic would warm the fastest, whereas the Antarctic is insulated by the circumpolar winds and ozone depletion. Note that the un-insulated Antarctic peninsula, is one of the fastest warmest parts of the world. We have always known that New Zealand, because of its isolation from large land-masses, would not warm as quickly as temperate continents.
With surface temperatures now matching closely with the models, the obfuscation has had to shift to the mid-troposphere, where there is still much to understand and measure. Natural variation may give the impression of a temporary hiatus, but the trend continues. Especially so now that the Pacific Decadal Oscillation has flipped over to a positive phase.
The Arctic is of course the Great White Warm Hope, but not going so well ………..
‘Global Warming Expedition Stopped In Its Tracks By Arctic Sea Ice’
http://dailycaller.com/2016/07/20/global-warming-expedition-stopped-in-its-tracks-by-arctic-sea-ice/
# # #
They are not in the Arctic at this juncture, currently stuck in Murmansk.
Pictured is another ‘Ship of Fools’, the MV Akademik Shokalskiy stranded in ice in Antarctica, December 29, 2013.
Remember Chris Turney?
Simon
>“The global mean is meaningless.” What do you mean? It is a useful metric.
For what?
The “global mean” doesn’t exist anywhere on earth. It is a totally meaningless metric.
>”The important thing is that reality is panning out just as the models predicted.”
What utter rubbish Simon. Just look at Schmidt’s graph, or any other of obs vs models graphs e.g. HadCRUT4 vs models (see below). The models are TOO WARM.
>”Note that the un-insulated Antarctic peninsula, is one of the fastest warmest parts of the world”
‘After warming fast, part of Antarctica gets a chill – study’
http://www.stuff.co.nz/world/82321379/after-warming-fast-part-of-antarctica-gets-a-chill–study
>”With surface temperatures now matching closely with the models”
Simon, just repeating an untruth doesn’t make it true – and it makes you look ridiculous:
HadCRUT4 vs Models
https://bobtisdale.files.wordpress.com/2016/07/10-model-data-time-series-hadcrut4.png
Clearly, surface temperatures are NOT “now matching closely with the models”. Being RCP8.5 is irrelevant because the scenarios are indistinguishable at this early stage i.e. it doesn’t matter what scenario is graphed they all look the same at 2016.
The IPCC concurs in AR5 Chapter 9 Evaluation of Climate Models:
“111 out of 114 realizations show a GMST trend over 1998–2012 that is higher than the entire HadCRUT4 trend ensemble”
“Almost all CMIP5 historical simulations do not reproduce the observed recent warming hiatus.”
That’s unequivocal Simon. The models are TOO WARM. The IPCC even offer reasons why the models are TOO WARM, for example in Box 9.2:
As time goes on this is more and more the case because GHG forcing is increasing rapidly but the earth’s energy balance isn’t (and therefore surface temperature isn’t either).
This is the critical discrepancy Simon:
0.6 W.m-2 trendless – earth’s energy balance AR5 Chapter 2
2.33+ W.m-2 trending – theoretical anthropogenic forcing AR5 Chapter 10 (CO2 1.9 W.m-2 @ 400ppm).
Theory is 4x observations.
Man-made climate change theory is wrong Simon. That’s all there is to it.
Simon
>”Natural variation may give the impression of a temporary hiatus, but the trend continues”
By what trend technique does “the trend continue”?
Extrinsic e.g. statistically inappropriate (i.e. not representative of the data) linear analysis?
Or,
Intrinsic e.g. Singular Spectral Analysis (SSA) or Empirical Mode Decomposition (EMD)?
Extrinsic is an externally imposed technique. Intrinsic is the inherent data signal. A curve (e.g. polynomial) is an extrinsic technique as is linear regression but a curve represents the temperature data better by statistical criteria.
But I actually agree with you Simon. Yes, the “the trend continues”, but trend of what?
Upthread I’ve shown that it is necessary to subtract MDV from GMST before comparing to the model mean.
ST = GMST – MDV
The residual is the secular trend (ST – a curve) which looks nothing like the trajectory of the data. The trajectory of 21st century GMST data is flat but the secular trend certainly is NOT flat (see Macias et al below).
Problem for MMCC theory is:
A) The secular trend (ST) in GMST now has a negative inflexion i.e. increasing CO2 cannot be the driver.
B) The CO2-forced model mean does NOT conform to the secular trend (ST) in GMST – the model mean is TOO WARM.
The Man-Made Climate Change theory is busted Simon.
**************************************************************************************
‘Application of the Singular Spectrum Analysis Technique to Study the Recent Hiatus on the Global Surface Temperature Record’
Diego Macias, Adolf Stips, Elisa Garcia-Gorriz (2014)
Abstract
Global surface temperature has been increasing since the beginning of the 20th century but with a highly variable warming rate, and the alternation of rapid warming periods with ‘hiatus’ decades is a constant throughout the series. The superimposition of a secular warming trend with natural multidecadal variability is the most accepted explanation for such a pattern. Since the start of the 21st century, the surface global mean temperature has not risen at the same rate as the top-of-atmosphere radiative energy input or greenhouse gas emissions, provoking scientific and social interest in determining the causes of this apparent discrepancy.
Multidecadal natural variability is the most commonly proposed cause for the present hiatus period. Here, we analyze the HadCRUT4 surface temperature database with spectral techniques to separate a multidecadal oscillation (MDV) from a secular trend (ST). Both signals combined account for nearly 88% of the total variability of the temperature series showing the main acceleration/deceleration periods already described elsewhere. Three stalling periods with very little warming could be found within the series, from 1878 to 1907, from 1945 to 1969 and from 2001 to the end of the series, all of them coincided with a cooling phase of the MDV. Henceforth, MDV seems to be the main cause of the different hiatus periods shown by the global surface temperature records.
However, and contrary to the two previous events, during the current hiatus period, the ST shows a strong fluctuation on the warming rate, with a large acceleration (0.0085°C year−1 to 0.017°C year−1) during 1992–2001 and a sharp deceleration (0.017°C year−1 to 0.003°C year−1) from 2002 onwards. This is the first time in the observational record that the ST shows such variability, so determining the causes and consequences of this change of behavior needs to be addressed by the scientific community.
Full paper
http://journals.plos.org/plosone/article?id=10.1371/journal.pone.0107222
[Macias et al] >”Since the start of the 21st century, the surface global mean temperature has not risen at the same rate as the top-of-atmosphere radiative energy input or greenhouse gas emissions, provoking scientific and social interest in determining the causes of this apparent discrepancy.”
By “top-of-atmosphere radiative energy input” they are referring to radiative forcing theory i.e. the theoretical effective anthropogenic radiative forcing (ERF) was 2.33 W.m-2 at the time of AR5 and increasing.
But neither the earth’s energy balance nor surface temperature is increasing as MMCC theory predicts as a result of a theoretical ERF of 2.33+ W.m-2:
0.6 W.m-2 trendless – earth’s energy balance AR5 Chapter 2
2.33+ W.m-2 trending – theoretical anthropogenic forcing AR5 Chapter 10 (CO2 1.9 W.m-2 @ 400ppm).
This is the critical discrepancy that falsifies the MMCC conjecture.
Simon,
This post describes the models overshooting observed temperatures and relates how Gavin Schmidt himself agrees with this. It falsifies the belief you express in this statement. Please either explain how you justify your belief, or retract the statement. As RC mentions, repeating an untruth doesn’t make it true, so how will you reconcile this?
Gary
>”That implies that that seasonality should be removed and the simplest way of doing that is to average over a year. The shape of the graph if all values were averaged over 12 months would show the underlying skewness applying to either pole.”
Agreed. That is exactly what Columbia University did in the graphs I linked too in the comment you are replying to:
Regional Changes – Zonal Means
Zonal mean, (a) 60-month and (b-d) 12-month running mean temperature changes in five zones: Arctic (90.0 – 64.2°N), N. Mid-Latitudes (64.2 – 23.6°N), Tropical (23.6°S), S. Mid-Latitudes (23.6 – 64.2°S), and Antarctic (64.2 – 90.0°S). (Data through June 2016 used. Updated on 2016/07/19, now with GHCN version 3.3.0 & ERSST v4)
http://www.columbia.edu/~mhs119/Temperature/T_moreFigs/ZonalT.gif
After the seasonal adjustment you require there’s a massive Arctic skew to the global mean. And a Northern Hemisphere skew too.
The global mean is irrelevant to the Southern Hemisphere excluding tropics (think Auckland, Sydney, Johannesburg, Buenos Aires) as demonstrated by GISTEMP below.
GISTEMP: Annual Mean Temperature Change for Three Latitude Bands [Graph]
http://data.giss.nasa.gov/gistemp/graphs_v3/Fig.B.pdf
Here’s the anomaly data:
Year Glob NHem SHem 24N-90N 24S-24N 90S-24S
2000 42 51 33 71 27 34
2001 55 64 45 80 44 44
2002 63 71 55 81 60 48
2003 62 72 51 80 63 41
2004 54 68 41 75 57 32
2005 69 84 54 98 64 46
2006 63 79 47 95 56 40
2007 66 83 48 108 47 48
2008 53 65 41 86 39 40
2009 64 70 58 73 68 50
2010 71 88 55 98 69 48
2011 60 71 49 92 37 58
2012 63 77 49 97 52 44
2013 65 76 55 87 58 53
2014 74 91 58 104 67 54
2015 87 113 60 125 92 41
http://data.giss.nasa.gov/gistemp/graphs_v3/Fig.B.txt
In 2015, 24N-90N (125) and 90S-24S (41).
NH excluding tropics is 3x SH excluding tropics. And not once is SHem anywhere near Glob.
“Glob” is completely meaningless. The closest to Glob in 2015 (87) is the Tropics (92) but look at 2000. Glob is 42, Tropics is 27.
Simon
>”Natural variation may give the impression of a temporary hiatus, but the trend continues. Especially so now that the Pacific Decadal Oscillation has flipped over to a positive phase”
Do you really know what you are talking about Simon?
A positive PDO does not necessarily mean GMST warming and a positive phase of natural multidecadal variation (MDV). 2000 was max positive MDV. 2015 was MDV-neutral. From 2015 to 2030 MDV will be in negative phase (see Macias et al upthread). So to reproduce the GMST profile from 2015 to 2030 starting from ST, MDV must be subtracted from ST:
ST – MDV = GMST
From 2015 until ST peaks, GMST will remain flatish i.e. ST will be ABOVE the GMST profile. After ST peaks GMST will go into cooling phase unless the MMCC is proved correct (doubtful) because ST will be cooling and it has more long-term effect on GMST than the oscillatory MDV does.
Question is: when will ST peak?
The solar conjecture incorporates planetary thermal lag via the oceanic heat sink i.e. we have to wait for an atmospheric temperature response to solar change, it is not instantaneous. Solar change commenced in the mid 2000s (PMOD) so if we add 20 years lag say (from various studies but certainly not definitive), we get an ST peak around 2025.
So the acid test for MMCC surface temperatures is the next 3 – 7 years. The acid test for the solar-centric conjecture doesn’t begin until the mid 2020s.
If you really want to invoke the PDO as a climate driver Simon, you will have to accept this:
A near perfect correlation of natural drivers with GMST versus a poor correlation of CO2 with GMST. And if you add in a CO2 component to PDO+AMO+SSI you will overshoot, As have the IPCC climate modelers.
Richard C>”The global mean is irrelevant to the Southern Hemisphere excluding tropics (think Auckland, Sydney, Johannesburg, Buenos Aires) as demonstrated by GISTEMP below.”
Actually, a global mean is irrelevant to any particular point somewhere on the globe! Which is to the point I was making about being circumspect about how it is to be used. I commented that it may be useful to have some sort of number for comparison purposes, but I would hesitate to give it any importance as a “metric”, which Simon considers appropriate. When noise is around ±0.5ºC differences of, say, ±0.2ºC do not convey much in the way of meaning.
One of the obfuscation techniques commonly in use is to display the information as “anomalies”. Everyone would be well advised to plot the information in actual values of, say, ºC with the origin set at 0º. Just eyeballing the data will give even the most casual viewer some perspective. That perspective is even better appreciated when considering a typical diurnal variation.
Should be:
“So to reproduce the GMST profile from 2015 to [2045] starting from ST, MDV must be subtracted from ST: ST – MDV = GMST [2015 – 2045]”
The equations over the MDV cycle are:
2045 neutral
2030 < MDV max negative ST – MDV = GMST
2015 neutral
2000 < MDV max positive ST + MDV = GMST
1985 neutral
1970 < MDV max negative ST – MDV = GMST
1955 neutral
1940 < MDV max positive ST + MDV = GMST
1925 neutral
1910 < MDV max negative ST – MDV = GMST
1895 neutral
Schmidt clarifies:
He’s got a problem looming with that though, his own GISTEMP Monthly shows GMST is plummeting back to neutral.
GISTEMP: Global Monthly Mean Surface Temperature Change
http://data.giss.nasa.gov/gistemp/graphs_v3/Fig.C.pdf
The June anomaly is unremarkable. After this a La Nina.
This post describes the models overshooting observed temperatures over a period of 15 years in the tropical mid-troposphere, i.e. 10 km above us. It is unclear whether this is natural variation, model inaccuracy, measurement error, or a combination of all three. 2016 will be interesting as I suspect that will be somewhere well within the models’ envelope. Also note Gavin’s point, which you ignored, that if the models were retrospectively initialised with now known realised forcing, the models’ projections would have been lower.
Gary
[You] >”Did you and Andy look at all months, or just February?”
[Me] >”No unfortunately. I picked up that graph from a comment thread somewhere. I don’t where to access the original graphs. I know there are similar graphs of each month because I saw another one recently of May I think it was but I didn’t save the link. I wish I knew who produces them. It’s not GISS I don’t think”
I remember now. You can generate your own from a GISS page but not from GISTEMP. June is set up to be generated here:
GISS Surface Temperature Analysis – Global Maps from GHCN v3 Data
http://data.giss.nasa.gov/gistemp/maps/
Just click ‘Make Map’ and scroll down to “Get the zonal means plot as PDF, PNG, or PostScript file”
PNG graphs
June 2016 Zonal Mean
http://data.giss.nasa.gov/tmp/gistemp/NMAPS/tmp_GHCN_GISS_ERSSTv4_1200km_Anom6_2016_2016_1951_1980_100__180_90_0__2_/amaps_zonal.png
February 2916 Zonal Mean
http://data.giss.nasa.gov/tmp/gistemp/NMAPS/tmp_GHCN_GISS_ERSSTv4_1200km_Anom2_2016_2016_1951_1980_100__180_90_0__2_/amaps_zonal.png
These graphs show the real GMST story by latitudinal zone.
Credit to where I got to that GISS zonal anomaly generator. A warmy blogger, Robert Scribbler, has been posting the graphs at his website: robertscribbler https://robertscribbler.com/
May 2016 Zonal Anomalies
https://robertscribbler.com/2016/06/13/may-marks-8th-consecutive-record-hot-month-in-nasas-global-temperature-measure/nasa-zonal-anomalies-may-2016/
June 2016 Zonal Anomalies
https://robertscribbler.com/2016/07/19/2016-global-heat-leaves-20th-century-temps-far-behind-june-another-hottest-month-on-record/june-zonal-anomalies/
Scribbler has this post:
‘Rapid Polar Warming Kicks ENSO Out of Climate Driver’s Seat, Sets off Big 2014-2016 Global Temperature Spike’
https://robertscribbler.com/2016/06/17/rapid-polar-warming-kicks-enso-out-of-the-climate-drivers-seat-sets-off-big-2014-2016-global-temperature-spike/
Read Arctic for”Polar”. I actually agree with this except now the “Rapid Arctic Warming” has dissipated. It turned into Rapid Arctic Cooling. Compare February Zonal Mean to June Zonal Mean side by side.
Simon,
We know the models got it wrong; the point you ignore is that Gavin agrees they got it wrong. The models are the only source for predictions of dangerous warming to come—but we need not now believe them, as they’re inaccurate. You had the same suspicion about model skill in many previous years, I would guess; but it doesn’t help us. I didn’t address Gavin’s point about rerunning the models with “now known” data because I thought it was too silly for words. It’s fairly easy to tweak a model to match past temperature; the point is to teach it to forecast the future.
Simon
<"This post describes the models overshooting observed temperatures over a period of 15 years in the tropical mid-troposphere, i.e. 10 km above us. It is unclear whether this is natural variation, model inaccuracy, measurement error, or a combination of all three."
Wrong at surface too. You might read the IPCC Chapter 9 quote upthread Simon. Here's their reasoning:
>”2016 will be interesting as I suspect that will be somewhere well within the models’ envelope.”
Good luck with that Simon. RSS is plummeting out of the envelope:
RSS from 2005
http://woodfortrees.org/plot/rss/from:2005
>”Also note Gavin’s point, which you ignored, that if the models were retrospectively initialised with now known realised forcing, the models’ projections would have been lower.”
Yes, EXAVTLY Simon. The IPCC agrees totally that the models are WRONG. They give 3 reasons as quoted above: (a) (b) and (c). They have neglected natural variation (a). Incorrect theoretical forcing (b) doesn’t seem to be the problem. That leaves model response error (c) in combination with neglected natural variation (a):
The model projections are TOO WARM because (a) natural variation has been neglected and (c) too much CO2 forcing is creating TOO MUCH HEAT i.e. CO2 forcing is at least excessive.
By the mid 2020s we will know if CO2 forcing is superfluous.
Simon
[You] >”Also note Gavin’s point, which you ignored, that if the models were retrospectively initialised with now known realised forcing, the models’ projections would have been lower.”
[Me] >”Incorrect theoretical forcing (b) doesn’t seem to be the problem”
Here’s what the IPCC has to say about (b) theoretical Radiative Forcing:
In respect to radiative forcing, supports my statement above but not Schmidt – “there are no apparent incorrect or missing global mean forcings in the CMIP5 models over the last 15 years that could explain the model–observations difference during the warming hiatus”.
That just leaves (a) natural variation and (b) model response error. The IPCC say their radiative forcing theory (a) is OK.
June’s GISTemp measures are now out. Despite the drop in temperature, June 2016 is still by far the warmest June ever recorded.
Simon
>”June’s GISTemp measures are now out. Despite the drop in temperature, June 2016 is still by far the warmest June ever recorded.”
“By far”? That Hot Whopper graph is wrong. June 2016 is unremarkable. LOTI is only 0.02 warmer than June 1998:
Monthly Mean Surface Temperature Anomaly (C)
——————————————–
Year+Month Station Land+Ocean
2016.04 1.36 1.14 January …..[ Hot Whopper gets this right ]
2016.13 1.64 1.33 February …..[ Hot Whopper says about 1.24 – WRONG ]
2016.21 1.62 1.28 March …..[ Hot Whopper gets this about right ]
2016.29 1.36 1.09 April …..[ Hot Whopper says about 1.22 – WRONG ]
2016.38 1.18 0.93 May …..[ Hot Whopper says about 1.16 – WRONG ]
2016.46 0.94 0.79 June …..[ Hot Whopper says about 1.1 – WRONG ]
1998.46 1.03 0.77 June …..[ Hot Whopper says about 0.7 – WRONG ]
http://data.giss.nasa.gov/gistemp/graphs_v3/Fig.C.txt
By station, June 2016 is cooler than June 1998 by 0.09.
Here’s a tip Simon: Hot Whopper is not a credible source. If you want GISS graphs and data go to GISS or someone whot knows how to plot graphs correctly.
IPCC>”This difference between simulated and observed trends could be caused by some combination of (a) internal climate variability, (b) missing or incorrect radiative forcing and (c) model response error.” (cited by Richard C)
This is fascinating. A semantic analysis might suggest:
(a)Internal climate variability: We haven’t been able to describe, or haven’t wished to include, all the physics.
(b)Missing or incorrect radiative forcing: We haven’t been able to correctly describe the AGW hypothesis.
(c)Model response error: We haven’t been able to force the assumptions and regression predictors and/or whatever to give us the answer we want.
Gary >”(b)Missing or incorrect radiative forcing: We haven’t been able to correctly describe the AGW hypothesis.”
They looked at that but decided they’d got their forcings right. From IPCC Chapter 9 (b) Radiative Forcing quote upthread:
So that just leaves (a) and (c) by their reasoning. But (b) and (c) are BOTH within their theoretical radiative forcing paradigm i.e. the models are responding (c) correctly to forcings (b) that don’t exist if their theory is wrong. They haven’t considered that very real possibility.
That their theory is wrong and the IPCC haven’t addressed the issue is laid out here:
IPCC Ignores IPCC Climate Change Criteria – Incompetence or Coverup?
https://dl.dropboxusercontent.com/u/52688456/IPCCIgnoresIPCCClimateChangeCriteria.pdf
And when the internal climate variability signal (a) is added in to the model mean profile as per the MDV signal extracted by Macias et al (see upthread), it makes the model mean profile even worse. MDV was max positive at 2000 but is missing from the models. Therefore the positive MDV signal must be added to the model mean over the period 1985 to 2015.
At 2015, MDV is neutral so the model mean stays as is at 2015 but is way too warm.
From 2015 to 2045, the MDV signal must be subtracted from the model mean but because the model mean is already too warm the subtraction still will not reconcile models to observations.
So it’s back to excessive anthro forcings in (b), contrary to their assessment, but that’s if their theory has any validity.
Their theory is falsified by the IPCC’s own primary climate change criteria (earth’s energy balance at TOA) as I’ve laid out in ‘IPCC Ignores IPCC Climate Change Criteria’ so the reason for the “model–observations difference” is superfluous anthro forcing – not just excessive, but superfluous, redundant.
Hot Whopper’s whopper:
OK so far, these numbers correspond to the NASA GISS anomaly data page upthread a bit and are unremarkable in respect to June 2016 compared to June 2015 and June 1998. But then she goes on:
This is the spin. She can’t crow about the June anomaly because it’s unremarkable compared to 2015 and 1998. NASA GISS resorts to the same spin for the same reasons:
What they don’t say of course is that the record was only broken by 0.01 (2015) and 0.02 (1998) i.e. nothing to crow about. So on with the spin – “The six-month period from January to June……….”.
That’s all they’ve got to crow about and all Sou at Hot Whopper can agonize over. So now back to the graph Simon posted, provenance Hot Whopper:
This is both misleading and meaningless spin. Poor gullible serially wrong Simon swallowed it hook line and sinker.
The last point June on Sou’s graph (1.1), which is the average of all months to date including June, means absolutely nothing if the actual anomaly for June has plummeted (0.79).
Even worse, by station June 2016 is cooler than June 1998 by 0.09.
Herein lies the perils of believing spin, and meaningless concocted graphs
GISS and Sou have different values for their 6 month average:
GISS – “The six-month period from January to June was also the planet’s warmest half-year on record, with an average temperature 1.3 degrees Celsius”
Sou – “The average for the six months to the end of June is 1.09 °C”
They are both correct but GISS zeal trumps Sou’s. GISS have averaged the Station data – NOT the LOTI data that Sou averaged:
GISS: Monthly Mean Surface Temperature Anomaly (C)
Year+Month Station Land+Ocean
2016.04 1.36 1.14 January
2016.13 1.64 1.33 February
2016.21 1.62 1.28 March
2016.29 1.36 1.09 April
2016.38 1.18 0.93 May
2016.46 0.94 0.79 June
2015.46 0.84 0.78 June
1998.46 1.03 0.77 June
http://data.giss.nasa.gov/gistemp/graphs_v3/Fig.C.txt
The lesson being: If you are going to resort to spin, you MUST choose the data that spins best.
Sou still has much to learn from GISS about spin apparently.
If Sou is going to plot “the average of the year to that month” (and neglect to tell anyone in the graph title), then she would have to plot the average of January to June at March/April – not June.
The average of January – June occurs at March/April.
This is no different to the IPCC’s Assessment Report prediction baseline as quoted by NIWA, T&T, and whoever else. Their baseline is the average of 1980 to 1999 data nominally centred on 1990 – not 1999.
Scurrilous Sou.
By June, Sou has a 6 month TRAILING average which she could then plot if she stipulated as such.
But her graph is NOT of “trailing averages”:
‘How do I Calculate a Trailing Average?’
http://www.ehow.com/how_6910909_do-calculate-trailing-average_.html
For January, the 6 month trailing average is 0.99 but Sou plots “year to date” which is the January anomaly (1.14).
For February, Sou plots the average of January and February – NOT the 6 month trailing average.
For March, Sou plots the average of January February and March – NOT the 6 month trailling average.
And so on.
Sou has 6 unrelated graph datapoints:
1 month trialling average (January)
2 month trailing average (February)
3 month trailing average (March)
4 month trailing average (April)
5 month trailing average (May)
6 month trailing average (June)
Sou plots all of those 6 trailing averages on a line graph and doesn’t stipulate on the title or the x axis what the datapoints actually are. The months on the x axis MUST also have the stipulation of the respective period of trailing average. For example:
April 4 month trailing average
And the value should be represented by a vertical bar graph because April is unrelated to the other months i.e. all of the months are in respect to different trailing averages
Lies, damn lies, and Sou’s graphs.
Antarctic Peninsula Has Been Cooling For Almost 20 Years, Scientists Confirm
https://notalotofpeopleknowthat.wordpress.com/2016/07/21/antarctic-peninsula-has-been-cooling-for-almost-20-years-scientists-confirm/
Richard C (NZ) on July 21, 2016 at 10:20 pm said:
>”That just leaves (a) natural variation and (b) model response error. The IPCC say their radiative forcing theory (a) is OK.”
Got it horribly wrong to. Should be:
“That just leaves (a) natural variation and [(c)] model response error. The IPCC say their radiative forcing theory [(b)] is OK.”
What Schmidt actually said about incorrect forcing in regard to TMT:
Qualitatively but why not quantitatively?
Reason: “This result is not simply transferable to the TMT record” i.e. it does not necessarily follow – a non sequitur.
The hotlink leads to this article:
‘Reconciling warming trends’
Gavin A. Schmidt, Drew T. Shindell and Kostas Tsigaridis
http://www.blc.arizona.edu/courses/schaffer/182h/Climate/Reconciling%20Warming%20Trends.pdf
Lots of problems with this which I’ll revisit over the weekend but for starters:
1) “Iinternal variability unrelated to ENSO” is MDV which is a greater factor by far than ENSO is. When an MDV signal is added in to the models (as it will eventually be conceded – give them time) that throws out all their reasoning completely.
Upthread I’ve posted Jeff Patterson’s ‘A TSI-Driven (solar) Climate Model’:
https://www.climateconversation.org.nz/2016/07/gavin-schmidt-confirms-model-excursions/comment-page-1/#comment-1500326
Jeff’s model goes a long way towards modeling Multi-Decadal Variation/Oscillation (MDV/MDO) that Schmidt et al haven’t even started on.
2) “if ENSO in each model had been in phase with observations”. Well yes, “IF”. But the models don’t do ENSO that can be compared to observations as Schmidt concedes. They have to add in known ENSO just as they would known MDV (when they get around to that). They also add in known transitory volcanic activity.
They are not really modeling when much of their “adjusted” model is just adding in known observations. The alternative is to neglect temporary volcanics, neglect temporary ENSO, neglect MDV and compare their model to observations with MDV removed and smoothed to eliminate ENSO which is really just “noise”. The volcanic influence in observations being only temporary and washes out relatively quickly..
3) “the observed trend matches the adjusted simulated temperature increase even more closely (Fig. 1b).”
No it doesn’t. The simulation is missing the MDV signal present in the observations. Conversely, the secular trend (ST) in the observations, which has MDV removed, is nothing like the model mean except that is is also a smooth curve but it is well below the model mean over the 21st century and coincides with MDV-neutral ENSO-neutral observations at 2015. At this date, the “adjusted” model mean is still well above the observations ST.
4) “may explain most of the discrepancy”. Read – “We’re speculating a lot, but we still don’t know for sure what’s going on. Sorry”
>”The simulation is missing the MDV signal present in the observations. Conversely, the secular trend (ST) in the observations, which has MDV removed, is nothing like the model mean except that is also a smooth curve but it is well below the model mean over the 21st century and coincides with MDV-neutral ENSO-neutral observations at 2015. At this date, the “adjusted” model mean is still well above the observations ST.”
Best seen in Macias et al Figure 1:
Figure 1. SSA reconstructed signals from HadCRUT4 global surface temperature anomalies.
http://journals.plos.org/plosone/article/figure/image?download&size=large&id=info:doi/10.1371/journal.pone.0107222.g001
The secular trend (ST, red line) is directly comparable to the model mean (not shown). At 2000 the ST (red line) is well below reconstructed GMST (thick black line). The model mean is above the GMST reconstruction (thick black line). Therefore the models are performing rather more badly than Schmidt, Shindell, and Tsigaridis think and even worse than most sceptics think.
Also easy to see that the ST (red line) is the MDV-neutral “spline” about which the GMST reconstruction (thick black line) oscillates. MDV-neutral is where the GMST reconstruction (thick black line) crosses the ST (red line).
2045 neutral
2030 < MDV max negative ST – MDV = GMST
2015 neutral
2000 < MDV max positive ST + MDV = GMST
1985 neutral
1970 < MDV max negative ST – MDV = GMST
1955 neutral
1940 < MDV max positive ST + MDV = GMST
1925 neutral
1910 < MDV max negative ST – MDV = GMST
1895 neutral
The model mean tracks the ST (red line) up until MDV-neutral 1955. Not too bad either at MDV-neutral 1985 although hard to make a judgment given the retroactively added volcanic activity to the models. But after that the model mean departs from the ST (red line) and veers wildly off too warm. This is the effect of theoretical anthropogenic forcing.
Jeff Patterson has a lot of the MDV issue figured out in his 'TSI-Driven (solar) Climate Model’ linked in previous comment. Most sceptics are oblivious to it all so there's a long way to go for them before understanding sets in (if ever).
Question is though: How long before Schmidt, Shindell, Tsigaridis, and all the other IPCC climate modelers figure it out?
>”Jeff Patterson has a lot of the MDV issue figured out in his ‘TSI-Driven (solar) Climate Model’ linked in previous comment.”
>”How long before Schmidt, Shindell, Tsigaridis, and all the other IPCC climate modelers figure it out?”
The IPCC are particularly clueless. Their “natural forcings only” simulations are laughable. Compare Jeff Patterson’s natural only model to the IPCC’s natural only models from Chapter 10 Detection and Attribution:
Patterson Natural Only: Modeled vs Observed
https://wattsupwiththat.files.wordpress.com/2016/02/image24.png
IPCC Natural Only: Modeled vs Observed (b)
http://www.ipcc.ch/report/graphics/images/Assessment%20Reports/AR5%20-%20WG1/Chapter%2010/Fig10-01.jpg
The IPCC then go on to conclude anthropogenic attribution from their hopelessly inadequate effort.
Patterson on the other hand, doesn’t need to invoke GHG forcing as as input parameterization. On the contrary, he models CO2 as an OUTPUT after getting temperature right.
Patterson:
[1] Singular Spectrum Analysis
Detecting the AGW Needle in the SST Haystack – Patterson
[2] Harmonic Decomposition
Digital Signal Processing analysis of global temperature data time series suggests global cooling ahead – Patterson
[3] Loess Filtering
Modelling Current Temperature Trends – Terence C. Mills, Loughborough University
[4] Windowed Regression
Changepoint analysis as applied to the surface temperature record – Patterson
# # #
None of these signal analysis techniques are employed by the IPCC in their Detection and Attribution.
Easy to see why they wouldn’t want attention drawn to them either.
Schmidt, Shindell, and Tsigaridis Figure 1:
“Interannual variability” ? Whoop-de-doo.
They adjust for a bit of noise but neglect multidecadal variabilty (MDV) with a cycle of around 60 years.
They’re so fixated on noise that they can’t see the signals.
They can’t see the wood for all the trees in the way.
Sorry Richard C that I haven’t been back regarding what you’ve posted since my last comment. Some medical problems got in the way yesterday which put paid to any activity!
My comment with a “semantic analysis” was meant to be sarcastic, and much of what you found and posted reinforces my comments. Rather curiously, I think. When a document starts to over-explain why there are deficiencies in models which purport to explain an hypothesis my antennae start to quiver. Others have described it as a bullshit metre. I wouldn’t, of course—I prefer a more refined statement. Much of it, and much of the information you followed it with have all the hallmarks of self-justification and desperation.
This discussion started a while back with the “Almost 100% of scientists …” statement from the Renwick and Naish road show and which I categorized as a fundamental need to have their stance vindicated by having others agree with them. This is, I believe, the rationale for trying to establish a “consensus”.
Richard C, the information you have posted on modelling is informative and interesting and the conclusions various authors have drawn offer an insight into what is, and what is not, possible.
In the far distant past (about 30 years ago) I had great success with linear modelling. That is where something can be described by linear relationships such as A + Bx1 + Cx2 + … for example I modeled the control of an alumina digester where two parallel trains of 12 shell-and-tube heat exchangers heated incoming caustic liquor flowed into three digesters into which bauxite slurry was injected. The stream out of the final digester passed through 12 flash tanks which supplied steam to the heat exchangers heating the incoming liquor. Each heat exchanger had 2 liquor temperatures and a pressure, a steam temperature and pressure totaling 6x12x2 or 144 variables, incoming liquor flow rate, 3 digester pressures, 3 digester temperatures, and the flow rate out of the final digester, 12 flow rates out of each of the flash tanks, and the 12 steam flow rates out of each of the flash tanks. That’s a total of 174 variables, most of which were measured or could be determined by energy or mass balances. I used a large linear matrix from which, every 5 minutes I calculated an inverted matrix and produced new predictors. I found I could predict the temperature distribution over the whole digestion unit to with ±1ºC up to 30 minutes ahead. We later built this into the control system.
The point about this is that a matrix of linear relationships can be employed very successfully in some circumstances without making any assumptions about physical relationships which may or may not exist between some of the parameters. If the technique is coupled with non-linear relationships such as those employed by the authors you cited above, it becomes a very useful tool, again without necessarily assuming any physical relations, let alone an hypothesis. It can also be a tool for examining potential relationships but it always has to bear remembered that such a model makes no assumptions and just because it may prove successful, as it did in my case, it does not imply any causal relationships.
Richard C > “They’re so fixated on noise that they can’t see the signals.”
Isn’t that the point? If the signal is buried in noise it is extremely difficult to extract it. As I understand signal processing, information (such as signals from deep space explorers) can be extracted from the noise because the useful information is associated with some sort of carrier signature which can be recognised by the processing system. If, however, the carrier signature is not known how can it be recognized and how can the information be extracted. I realise that this is the basis for a huge amount of research effort in signal processing and digital filtering but none-the-less I maintain that if you don’t know what you are looking for how will you recognize it when you see it? Wasn’t this part of the problem with SETI?
Gary
>”f the signal is buried in noise it is extremely difficult to extract it”
Except the 2 major signals in GMST (which is overwhelmed by the Northern Hemisphere) are neither buried nor difficult to extract. On the contrary, they are staring everyone in the face and reasonably easy to extract with EMD (I’ve done this with HadSST3) and SSA (haven’t got to grips with this unfortunately).
The 2 major signals in GMST are the secular trend (ST) and multidecadal variation/oscillation (MDV/MDO) which has a period of about 60 years (the “60 year Climate Cycle”). Once these signals are extracted, they can then be added to reconstruct noiseless GMST.
The best SSA example of this that I know of is Macias et al linked upthread. Here’s their paper, their Figure 1, and my commentary again:
‘Application of the Singular Spectrum Analysis Technique to Study the Recent Hiatus on the Global Surface Temperature Record’
Diego Macias, Adolf Stips, Elisa Garcia-Gorriz (2014)
Full paper: http://journals.plos.org/plosone/article?id=10.1371/journal.pone.0107222
Figure 1. SSA reconstructed signals from HadCRUT4 global surface temperature anomalies.
http://journals.plos.org/plosone/article/figure/image?download&size=large&id=info:doi/10.1371/journal.pone.0107222.g001
The secular trend (ST, red line) is directly comparable to the model mean (not shown). At 2000 the ST (red line) is well below reconstructed GMST (thick black line). The model mean is above the GMST reconstruction (thick black line). Therefore the models are performing rather more badly than Schmidt, Shindell, and Tsigaridis think and even worse than most sceptics think.
Also easy to see that the ST (red line) is the MDV-neutral “spline” about which the GMST reconstruction (thick black line) oscillates. MDV-neutral is where the GMST reconstruction (thick black line) crosses the ST (red line).
2045 neutral
2030 < MDV max negative ST – MDV = GMST
2015 neutral
2000 < MDV max positive ST + MDV = GMST
1985 neutral
1970 < MDV max negative ST – MDV = GMST
1955 neutral
1940 < MDV max positive ST + MDV = GMST
1925 neutral
1910 < MDV max negative ST – MDV = GMST
1895 neutral
The model mean tracks the ST (red line) up until MDV-neutral 1955. Not too bad either at MDV-neutral 1985 although hard to make a judgment given the retroactively added volcanic activity to the models. But after that the model mean departs from the ST (red line) and veers wildly off too warm. This is the effect of theoretical anthropogenic forcing.
# # #
Note these are nominal dates. It breaks down a bit around 1985 due to volcanic activity.
BTW #1. That was an impressive control model – out of my league by about a light year or two.
BTW #2. I had gut inflammation for years, lost a lot of weight and couldn't put it on because I couldn't digest food properly. Exacerbated by night shift work. Cured with anti-inflammatory foods. A persimmon a day had an immediate effect and I also drink a glass of grape juice a day. Note there is a difference between anti-inflammatory and anti-oxidant foods. Just at the end of persimmon season right now (I think) so you will only see end of season fruit in the shops – if any. But worth every cent at any price.
You have GOT to cure inflammation if you have it. Inflammation is the root of many diseases which in turn can lead to cancer.
>”BTW #2. I had gut inflammation for years, lost a lot of weight and couldn’t put it on because I couldn’t digest food properly. Exacerbated by night shift work.”
I’ve been wondering lately whether it was started by glyphosate (think Roundup), possibly in bread. This is the big controversy in Europe and North America, wheat growers in USA spray Roundup on the crop just before harvest as a desiccant. NZ gets wheat from Australia but I don’t know if there is the same practice in Australia or not.
Not sure where the issue has got to but here’s an article from April 15, 2014 for example:
‘Gut-Wrenching New Studies Reveal the Insidious Effects of Glyphosate’
Glyphosate, the active ingredient in Monsanto’s Roundup herbicide, is possibly “the most important factor in the development of multiple chronic diseases and conditions that have become prevalent in Westernized societies”
http://articles.mercola.com/sites/articles/archive/2014/04/15/glyphosate-health-effects.aspx
Richard C thanks for your concern and advice. Yesterday the pool at Porirua had to be closed because of 5 people infected with cryptosporidiosis. It appears to be going round the Wellington region and the symptoms exactly match what I have encountered over the last 10 days: stomach cramps & etc! The symptoms can last for up to 2 weeks so hopefully that is about over for me.
‘U.N. experts find weed killer glyphosate unlikely to cause cancer’ – May 16, 2016
The pesticide glyphosate, sold by Monsanto in its Roundup weed killer product and widely used in agriculture and by gardeners, is unlikely to cause cancer in people, according to a new safety review by United Nations health, agriculture and food experts.
In a statement likely to intensify a row over its potential health impact, experts from the U.N.’s Food and Agriculture Organization (FAO) and World Health Organization (WHO) said glyphosate is “unlikely to pose a carcinogenic risk to humans” exposed to it through food. It is mostly used on crops.
Having reviewed the scientific evidence, the joint WHO/FAO committee also said glyphosate is unlikely to be genotoxic in humans. In other words, it is not likely to have a destructive effect on cells’ genetic material.
http://www.reuters.com/article/us-health-who-glyphosate-idUSKCN0Y71HR
# # #
“Unlikely” is not very reassuring.
I think the danger, if any, is more insidious than as a direct cause of cancer.
Gary >”cryptosporidiosis. …….. symptoms ………..stomach cramps & etc!…….2 weeks”
Inflammatory bowel disease much the same but months and years not weeks. I had stomach cramps for 12 hours straight one day, everything cramped up and I couldn’t straighten up. I wouldn’t wish it on anyone.
I thought it was just night shifts but when Psa struck kiwifruit certain people in the industry had to have medical tests. That’s how I found out my condition eventually but I had it before night shifts when I thought about it. People were finding out conditions they never knew about from the Psa medical tests. I talked to a guy who was found to have prostate cancer he didn’t know about. He was in hospital the week after the test result. Saved his life.
Richard C we’re kinda straying away from the topic. There is a strong message coming through though. If you have a problem, don’t delay doing something about it. I lost a good friend a couple of weeks ago with some form of stomach cancer. He ignored it for years until the pain became too much. By then it was too late to do anything. Peter Williams, the QC and activist ignored prostate cancer until it was too late: probably cost him 10 years of life. My prostate was removed a couple of years ago after 10 years of close monitoring. PSA markers are now undetectable. My recent condition is exacerbated by a bout of anæmia which landed me in hospital for a week or so. Still don’t know what the problem was but I’m awaiting the results of a camera capsule examination. The wonders of modern technology!
Richard C, thanks for the reference on singular spectrum analysis. I’m working through it and trying to reconcile it with some things I know. What I don’t know is how they allocated certain meanings to the Eigenvalues. And I am still puzzling over their use of the term “secular”—although it may be appropriate in that proponents of AGW seem to have a “belief” or “faith” in the hypothesis that physics doesn’t seem to yield! I have found that UCLA has a program (an App) which will calculate the Eigenvalues and is implementable on a UNIX based system (various flavours of Linux, or Mac OS X version 10) which I am looking at with a view to installing it on my MacBook Pro. If I can successfully implement it I might try running the NIWA data on it (Raw and adjusted 7SS series) to see what we can get out of it. Might be worth a post, Richard T?
For what it is worth, although I have only just done some preliminary reading, it has a familiarity about it. 40 years ago I developed a method for endeavouring to analyse very noisy pressure measurements at the wall of a tube carrying a two-phase mixture of air and water. The technique I developed was based on a method of Hamming for analyzing discrete (i.e. digital) data in which autocorrelations were calculated after successive decimations of the data. In this case, decimation means removing every 10th value. I went a step further and applied a Fourier Analysis (using a Fast Fourier Transform) at each stage. In this way I managed to find the fundamental and some other frequencies in the mess of information. I suspect that the SSA is very similar. In those days we didn’t have the online search facilities we now have so I was unable to dig out some of the references cited in the paper you found, and I didn’t have the computational facilities to be able to play with the matrices in the way we now can. I look at my Masters Thesis from time to time and gawp at the maths I used then. I wouldn’t want to do it again! I couldn’t now—it is beyond me.
I’ll let you know how I get on, but don’t hold your breath.
Gary
I was hoping to pique your interest in SSA because you have the background and skills to go further with SSA than I can. I’ve looked at some of those packages including coding in R but have not gone any further. I saw that UCLA App listed too.
>And I am still puzzling over their use of the term “secular”
Just the more appropriate term for the inherent trend after all the noise and fluctuations are eliminated. In EMD it is the residual after all of the intermediate frequencies have ceased to be extracted e.g. rough example, IMFs 1, 2, 3 just noise, IMF 5 a minor oscillation, IMF 7 a major oscillation, last a residual. When new data is added to the series, eventually the residual becomes the last IMF (IMF 8 in example) and a new residual emerges. In other words, you cannot really “project” an EMD residual because after a period of time elapses you will have only projected an IMF.
I think there’s a similar situation with SSA i.e. you may have subjectively selected a spectral “window” in which you think the secular trend lies but new data may prove you wrong. Not sure at all about this though.
SSA is much different to EMD as I understand e.g. the SSA-M technique is a moving “window” of data the length of which the analyst selects from which a “single” spectrum is identified i.e. some human subjectivity is involved (EMD is completely objective). I may not have this quite right though.
In a climate time series the “secular trend” (ST) is just the “long-term trend” in the data sans any oscillatory components (e.g. MDV), fluctuations or noise. This is very important, the ST in GMST is certainly not linear as climate scientists seem to think and it is only the ST that has an apples-to-apples comparability to the CMIP model mean. Climate scientists (and most sceptics) can’t grasp this though.
There is also a very important fundamental difference between trend techniques – intrinsic vs extrinsic.
Intrinsic: Is extraction of the inherent data signal, In EMD there is complete analyst objectivity. In SSA there is an element of analyst subjectivity (I think) but an intrinsic exercise nonetheless.
Extrinsic: Is the external imposition of a trend on the data by an analyst assumption e.g. assuming the trend in fluctuating data is linear and determining the trend by linear regression. Similarly, assuming the trend in the data is a polynomial or is exponential an imposing the respective technique on the data.
I think the extrinsic assumption is one of the worst aspects prevalent in climate science, now that I’ve delved into it.
>”If I can successfully implement it I might try running the NIWA data on it (Raw and adjusted 7SS series) to see what we can get out of it”
May not be a long enough series to be of much use. I suggest BEST NZ monthly data. Pre 1970 it is a load of rubbish but at least you have a much longer series and it’s easy to get hold of the data at the BEST website.
I did an EMD exercise using the 7SS that Richard T posted (unsophisticated by your standards I’m guessing Gary):
NZ vs S. Hemisphere temperatures [EMD analysis vs Linear trend]
https://www.climateconversation.org.nz/2011/01/nz-vs-s-hemisphere-temperatures/
With a longer series the EMD residual I found would just be an IMF. It is VERY sensitive, note the Update.
>”Might be worth a post, Richard T?”
Gary, what I would really REALLY like to see is the ST in Southern Hemisphere HadCRUT4 versus the ST in Northern Hemisphere HadCRUT4. Or a combination of say NH vs SH CRUTEM4 and HadSST3
Take a look at these graphs:
HadSST3 NH vs CRUTEM4 NH vs HadSST3 SH vs CRUTEM4 SH (5 yr smoothing)
http://woodfortrees.org/plot/hadsst3nh/mean:60/plot/hadsst3sh/mean:60/plot/crutem4vnh/mean:60/plot/crutem4vsh/mean:60
Separately,
HadSST3 NH vs CRUTEM4 NH
http://woodfortrees.org/plot/hadsst3nh/mean:60/plot/crutem4vnh/mean:60
HadSST3 SH vs CRUTEM4 SH
http://woodfortrees.org/plot/hadsst3sh/mean:60/plot/crutem4vsh/mean:60
HadCRUT4 Land+Ocean is HadSST3+CRUTEM4 (assuming you weren’t quite clear about this maybe)
Obviously the secular trends in each respective series (4 of them) are all different. We’ve been used to looking at a globally averaged GMST but that is illusory. The Southern Hemisphere is primarily overwhelmed by Land in the Northern Hemisphere. Then it is overwhelmed by NH Ocean too. So the NH completely skews GMST into a meaningless metric.
Ultimately the comparison is with the ST in the CMIP model mean – THAT would be most revealing.
Two more interesting comparisons:
HadSST3 NH vs HadSST3 SH
http://woodfortrees.org/plot/hadsst3nh/mean:60/plot/hadsst3sh/mean:60
CRUTEM4 NH vs CRUTEM4 SH
http://woodfortrees.org/plot/crutem4vnh/mean:60/plot/crutem4vsh/mean:60
Ocean is similar but Land is radically different in each hemisphere.
I don’t how anyone can even consider “trying to tease out the signal of human-caused climate change” from all of this (as Micheal Mann puts it).
Richard C > “I was hoping to pique your interest in SSA ”
You’ll keep!
I have downloaded the package from UCLA: requires a Unix command to start it. I’ve also downloaded about 150 pages of notes to read. As I said, don’t hold your breath, this is going to take some time.
As to what I look at I think I will look at the 7SS series first.
Richard C, just to let you know what you have let me in for!
“SSA allows one to unravel the information embedded in the delay-coordinate phase space by decomposing the sequence of augmented vectors thus obtained into elementary patterns of behaviour. It does so by providing data-adaptive filters that help separate the time series into components that are statistically independent, at zero lag, in the augmented vector space of interest. These components can be classified essentially into trends, oscillatory patterns, and noise. As we shall see, it is an important feature of SSA that the trends need not be linear and that the oscillations can be amplitude and phase modulated.”
http://research.atmos.ucla.edu/tcd//PREPRINTS/2000RG.pdf
Whew!
Gary >”Richard C, just to let you know what you have let me in for!”
Heh. How many PhDs’ were involved in producing that first sentence? Nuts. Now I will have to get some familiarity with that paper I suppose.
I see M-SSA in there beginning page 27:
More on M-SSA below because in the author list I see Michael E. Mann:
ADVANCED SPECTRAL METHODS FOR CLIMATIC TIME SERIES
M. Ghil, M. R. Allen, M. D. Dettinger, K. Ide, D. Kondrashov, M. E. Mann, A. W. Robertson, A. Saunders, Y. Tian, F. Varadi, and P. Yiou (2001)
Ok, so Mann's been on to this since before 2001 so why has he not been applying SSA appropriately since then in climate series analysis where it matters?
Wyatt and Curry 2014 (online 2013) employed M-SSA to examine aspects of their hypothesized "Stadium Wave". The hypothesis underpinning the stadium-wave is that on long time scales, climate variability organizes into network behavior executed through coupled dynamics among ocean, ice, and atmospheric circulation patterns.
Mann et al (2014) took a different approach to the same study and say in effect that Wyatt and Curry's analysis and hypothesis is incorrect. Marcia Wyatt writes about the differences in approach here:
'Disentangling forced from intrinsic climate variability'
Posted on July 9, 2014 | by Marcia Wyatt
https://judithcurry.com/2014/07/09/disentangling-forced-from-intrinsic-climate-variability/
In response to Mann et al (2014), Sergey Kravtsov, Marcia Wyatt, Judith Curry, and Anastasios Tsonis produced a paper, Kravtsov et al (2014), which further investigates the stadium wave hypothesis again employing M-SSA. Curry and Wyatt write about it here:
'Two contrasting views of multidecadal climate variability in the 20th century'
Posted on September 28, 2014 | by Judith Curry
https://judithcurry.com/2014/09/28/two-contrasting-views-of-multidecadal-climate-variability-in-the-20th-century/
In that post Wyatt writes:
Thing is: Mann was at the forefront of the use of M-SSA but did not employ it in Mann et al (2014). But Wyatt and Curry (2014) and subsequently Kravtsov et al (2014) did employ M-SSA. The Mann et al challenge to Wyatt and Curry makes no recourse to M-SSA.
So why did Mann pioneer ADVANCED SPECTRAL METHODS FOR CLIMATIC TIME SERIES but then not employ them?
Given Wyatt’s account of Mann et al (2014), it seems to me that Mann does not like the results of M-SSA because he has discovered those results disallow his belief that the variability in question is radiatively forced and thence an opportunity for anthropogenic attribution. Marcia Wyatt puts it this way in ‘Disentangling forced from intrinsic climate variability’
You have to wonder why Mann first embraced the M-SSA technique but then dropped it like a hot potato. I think I know why.
Gary.
Following on from the comment above involving Mann and how he will do anything to get the “right” answer. I’m reproducing a comment from a post earlier this year:
*********************************************************************
Richard C (NZ) on March 20, 2016 at 6:06 pm said:
Steve Sherwood and Stefan Rahmstorf at Hot Topic:
This is dead wrong. The hotlink is to this Rahmstorf Tweet:
https://twitter.com/rahmstorf/status/698380997222510592
Which contains this graph:
https://pbs.twimg.com/media/CbElthfUAAA1DVS.jpg
Graph looks dodgy. Reason becomes clear by following the link in the Tweet to this paper:
‘The Likelihood of Recent Record Warmth’
Michael E. Mann, Stefan Rahmstorf, Byron A. Steinman, Martin Tingley & Sonya K. Miller (2015)
http://www.nature.com/articles/srep19831
Yes you are looking at GISTEMP. No you are not looking at CMIP5 model output. You are looking at “adjusted” model output i.e. a residual.
The giveaway is this:
It is totally unnecessary to make recourse to climate models in order to eliminate “noise” from GMST e.g. minor fluctuations, ENSO activity, MDV. But Mann et al left GMST as-is, instead “adjusting” the model mean. This is bogus.
The model mean is ENSO-neutral and MDV-neutral. Therefore the model mean should have been left as-is and GMST “adjusted” i.e. a GMST residual, NOT a model mean residual. The model mean already IS a residual.
More>>>>>>>
https://www.climateconversation.org.nz/2016/02/met-office-shock-forecast-warming-to-continue/#comment-1448020
# # #
Mann et al (2015) could have used SSA to eliminate MDV form GMST in order to compare to the CMIP5 model mean. Instead, they have adjusted the model mean in order to reconcile models vs observations i.e. the “right” answer.
I hope the sheer bogosity of Mann et al (2015) does not escape you Gary.
Note that Rahmstorf Tweeting his bogus graph is not much different to Simon upthread posting the shonky Hot Whopper graph.
It’s the Warmy way apparently.
Latest reason the models are wrong (the models are right, the observations are wrong):
‘Historical Records Miss a Fifth of Global Warming: NASA’ – Jet Propulsion Laboratory July 21, 2016
A new NASA-led study [Richardson et al (2016)] finds that almost one-fifth of the global warming that has occurred in the past 150 years has been missed by historical records due to quirks in how global temperatures were recorded. The study explains why projections of future climate based solely on historical records estimate lower rates of warming than predictions from climate models.
The study applied the quirks in the historical records to climate model output and then performed the same calculations on both the models and the observations to make the first true apples-to-apples comparison of warming rates. With this modification, the models and observations largely agree on expected near-term global warming. The results were published in the journal Nature Climate Change. Mark Richardson of NASA’s Jet Propulsion Laboratory, Pasadena, California, is the lead author.
More>>>>
http://www.jpl.nasa.gov/news/news.php?feature=6576
# # #
What “largely agree” means remains to be seen (paywalled). The “Fifth of Global Warming” the observations “miss” (apparently), is Arctic warming only the models produce and some other minor warming that MUST have been there because the observations need to be adjusted to “account” for it.
Here’s the kicker:
The assumption, by circular reasoning, is this : The warming was there all along (we now “know” from our models – all the extra 19% of it) but it is only mainly in the Arctic, and we have to “tweak” the observations a bit too. If we neglect the Arctic and the Arctic warming in the models and “tweak” the observations, the models and observations will reconcile.
Question is though, what difference was there between the “limited coverage” models and the CMIP5 models? Again, this remains to be seen (paywalled)
Still problematic because the satellites have the coverage that surface measurements don’t including much of the Arctic region e.g. the Schmidt and Christy graphs in the post. The surface disparity that JPL’s Richardson et al is addressing began way back in the 1860s (apparently), but the major disparity between temperature derived from NASA’s own satellites and the models only began in the mid 1990s. Same at the surface although the divergence began after ab out 1985.
The CMIP5 models are spot on surface observations up until 1955. And still good at 1985 i.e. there was no reason to “adjust” either models or observations prior to 1985. If Richardson et al have “adjusted” both models and observations prior to 1985 then they have turned a very satisfactory situation into a problematic situation.
NASA’s JPL are digging themselves into a very big hole.
Climategate email:
“What if climate change appears to be just mainly a multidecadal natural fluctuation? They’ll kill us probably “
— Tommy Willis, Swansea University
Mann has figured in several references in the papers I have been reading. But I note that he does not appear to be a lead author in many of them. Which suggests to me that he has been lending his imprimatur to the papers without necessarily contributing much in a technical way. That is not to say that he isn’t well versed in the methods, just that he has not chosen to use them. That’s not an unusual situation. Anyway, I would have though that his tree ring analyses would not have lent themselves to this sort of analysis, and it wouldn’t be appropriate to attempt it on the time series he generated because that would have been subjected to assumed statistical processes.
Richard C > “The assumption, by circular reasoning, is this : The warming was there all along (we now “know” from our models – all the extra 19% of it) but it is only mainly in the Arctic, and we have to “tweak” the observations a bit too. If we neglect the Arctic and the Arctic warming in the models and “tweak” the observations, the models and observations will reconcile.”
This is an example of a logical fallacy, a direct analogy to the CO2/temperature relationship. post hoc ergo propter hoc: CO2 rises and temperature rises therefore CO2 rising caused the temperature to rise. But, of course, as we know, temperature rose first because man didn’t really start adding significant CO2 to the atmosphere until the 1950’s, or so we are told. Actually I rather like the idea that temperature leads CO2 by 800 years, so we can blame the MWP for the current increase in CO2.
By the way, Richard, I still dislike you! 100 pages of reading, a package that doesn’t work well because the graphics side is hard (at least for my feeble brain) to implement, and I’m likely to spend some considerable funds on another package I know will work (because I have purchased a much cheaper limited implementation). I guess you get what you pay for! But … it does look promising. What do you know about eigenvectors? 😉
Gary
>”I would have though that his tree ring analyses would not have lent themselves to this sort of analysis”
His recent opposition to Wyatt and Curry and subsequently Kravtsov, Wyatt, Curry, and Tsonis isn’t about tree rings though. His 2014 paper in response to Wyatt and curry is meteorological, climate, and oceanographic data as I understand without checking (but no tree rings I’m sure), and the signals they present. SSA more than lends itself to this sort of analysis as his opposition authors demonstrate (as do Macias et al). But Mann didn’t take that route because, I’m inclined to think, he would have to throw out his radiative forcing approach if he did. He can’t give that approach up because then he can’t make an anthro attribution because that’s the paradigm that gives him the opportunity – if he can work it in his favour. SSA (and EMD) extracts so many natural variation signals that there is no room left for an anthro signal, he can’t “tease” it out as he puts it.
And then following that, the Mann et al (2015) models vs observations of temperature paper is exactly the application that SSA is particularly suited in order to get to the respective comparative signals. My ending comment on that was this:
O/T
Dr Jarrod Gilbert: Why climate denial should be a criminal offence
http://www.nzherald.co.nz/nz/news/article.cfm?c_id=1&objectid=11681154
Dr Jarrod Gilbert is a sociologist at the University of Canterbury and the lead researcher at Independent Research Solutions. He is an award-winning writer who specialises in research with practical applications.
Perhaps this “sociologist” could suggest a suitable punishment for the criminal offense of denying the climate.
Execution by firing squad? 25 years in the clinker? Hanging? Electric chair? Lethal injection?
All offers are open.
Interesting that this gent has written a book on gangs in NZ
http://www.jarrodgilbert.com/
He’ll be well familiar with the gang at the University of Victoria then
Andy, this is a topic Richard T should explore in another post, and I have no doubt he probably will. He should be told that the RICO bandwagon in the US looks like it is losing its wheels. “Rats deserting sin king ships” comes to mind!
Richard C > “But it might be as you imply Gary, that Mann is not a technical exponent of SSA so he can’t use it. But he used principal components analysis (PCA) in his tree ring study so surely he could make the step to SSA?”
He is one of the authors of the seminal paper for the UCLA-developed paper I cited above http://research.atmos.ucla.edu/tcd//PREPRINTS/2000RG.pdf.
Gary
>”He [Mann] is one of the authors of the seminal paper for the UCLA-developed paper I cited above”
Yes I saw that immediately and noted it upthread here:
Richard C (NZ) on July 24, 2016 at 11:29 pm said:
https://www.climateconversation.org.nz/2016/07/gavin-schmidt-confirms-model-excursions/comment-page-1/#comment-1501514
That was what got me going on the Mann vs Wyatt & Curry/Kravtsov et al saga.
Re Dr Jarrod Gilbert: Why climate denial should be a criminal offence
Given Gilbert’s deference to the 97% consensus, this topic would have been better in the Naish and Renwick thread – but good to know Andy. I’ll link and reply to the Gilbert article in that thread.
Willis Eschenbach:
Precipitable Water
Figure 4. Decomposition of the total precipitable water data (upper panel) into the seasonal (middle panel) and residual (bottom panel) components.
https://wattsupwiththat.files.wordpress.com/2016/07/plotdecomp-total-precipitable-water-tpw.png?w=720&h=674
Some things of interest. First, in the bottom panel you can see the effect on TPW of the El Nino episodes in 1997/98, 2010/11, and 2015/16. You can also see that we haven’t quite recovered from the most recent episode.
Next, there is a clear trend in the TPW data. The total change over the period is ~ 1.5 kg/m^2, centered around the long-term mean of 28.7 kg/m^2.
And utilizing the relationship between water content and atmospheric absorption derived above, this indicates an increase in downwelling radiation of 3.3 W/m2 over the period.
This leads us to a curious position where we have had a larger change in forcing from water vapor since 1988 than from all the other IPCC-listed forcings since 1750 … so where is the corresponding warming?
https://wattsupwiththat.com/2016/07/25/precipitable-water/
# # #
Yes. Where?
Total of 5.6 W.m-2 but earth’s energy imbalance remains at 0.6 W.m-2.
Two papers that together falsify the Man-Made Climate Change Theory.
1) ‘An observationally based energy balance for the Earth since 1950’
D. M. Murphy, S. Solomon, R. W. Portmann, K. H. Rosenlof, P. M. Forster, T. Wong (2009)
http://onlinelibrary.wiley.com/doi/10.1029/2009JD012105/full
There is obviously a massive discrepancy between theoretical forcing and actual earth heating. Murphy et al describe the discrepancy as “striking”:
Murphy et al (2009) Figure 6
http://onlinelibrary.wiley.com/store/10.1029/2009JD012105/asset/image_n/jgrd15636-fig-0006.png?v=1&s=1d48ee59aed4b059a12eea9575028e88a7b134ce
Total cumulative theoretical forcing is 1700 x 10^21 Joules 1950 – 2004 of which 10% is 170 x 10^21 Joules.
In Figure 6, solar forcing is already 100 x 10^21 Joules leaving a residual of 70 x 10^21 Joules. Nordell and Gervet (2009) below estimate only 27.3 x 10^21 Joules (say 30 at 2004) actual energy accumulation total over the entire extended period 1880–2000 (say 2004):
2) ‘Global energy accumulation and net heat emission’ [1880–2000 ]
Bo Nordell and Bruno Gervet (2009)
http://www.ltu.se/cms_fs/1.5035!/nordell-gervet%20ijgw.pdf
So Murphy et al’s 1950 – 2004 earth heating is still 140 x 10^21 Joules MORE than Nordell and Gervet’s estimate for earth heating over the entire period 1880-2004 (170 vs 30 x 10^21 Joules) even after Murphy et al throw out almost all GhG forcing and a bit of solar forcing (90% total discard).
In other words, Murphy et al would have to discard ALL GhG forcing (and a bit more solar forcing) to reconcile with Nordell and Gervet.
It gets worse for the Man-Made Theory after the GhG discard:
Not specified but obviously Surface Solar Radiation (SSR) i.e. solar radiation reaching the surface through clouds, aerosols, etc, would drive “small changes in heat transfer into the ocean” along with windiness or changes in wind patterns.
Murphy et al (2009) together with Nordell and Gervet (2009) effectively falsify the Man-Made Climate Change Theory.
From my Richardson et al (2016) comment
>”Still problematic because the satellites have the coverage that surface measurements don’t including much of the Arctic region e.g. the Schmidt and Christy graphs in the post.”
Not quite right here. Only the Christy graph is “Global”. Schmidt’s is only Tropics.
Is Climate Too Complex to Model or Predict? Scientists say no
By Seth B. Darling & Douglas L. Sisterson, posted Jul 27th, 2016
Seth B. Darling is a scientist at the Argonne National Laboratory, specializing in energy and water. Douglas L. Sisterson is a senior manager at the Argonne National Laboratory.
http://www.popsci.com/is-climate-too-complex-to-model-or-predict
Hidden away in the very long screed (no graphs) is this:
The “rare counterexample” just happens to be at the time of the highest fossil fuel emissions in the entire industrial era.
So no, “this sort of thing will [NOT] make the skeptic stop saying that the IPCC is exaggerating the problem”.
On the contrary.